As a parent of three children under 4, I was hit hard by last month's announcement that the Food and Drug Administration was delaying its review of Pfizer-BioNTech's Covid-19 vaccine for children under 5.
Like many caregivers guarding young children against the coronavirus, my winter has been full of rapid tests, mask reorders and outdoor play dates in borderline frostbite conditions. I'm able to manage this because I believe it's temporary; we just need to hold out a little longer until our children can get vaccinated.
But because I study statistics, I'm also racked with concern that if the data had been assessed in a more nuanced way, we might be putting vaccination appointments on the family calendar right now.
It's unclear why the FDA paused the review. The most recent data hasn't been shared, and reporting suggests Pfizer found that the omicron wave led to many more infections than previously seen in its clinical trial. The decision was made to wait for data on the third dose. Perhaps the two doses were not effective enough for the full group, though earlier data had suggested the vaccines produced a desired immune response for children ages 6 months to 24 months.
The bigger issue, as I see it, is in general statistical methods that are often relied on to evaluate the effectiveness of vaccines and drugs. The standard approach used in almost all clinical trials and endorsed by the FDA requires new drugs to meet an arbitrary statistical threshold, the one people who have taken stats classes may recognize as statistical significance. This is appealing because it serves as a standardized final exam that experimental results all have to pass, unaided by preconceptions on the part of the reviewers or special pleading by the experimenters.
But the whole idea of statistical significance has been losing favor among many statisticians, for two good reasons. For one, this thinking is inherently binary; after the number crunching is complete, results are classified as significant or not significant, suggesting a finality and certitude that are rarely justified, and second, like any standardized test, it's overly reductive. If relied on too heavily, it becomes a substitute for a more thoughtful, holistic analysis of the data, including important scientific context.
Nearly three years ago, an open letter signed by more than 800 scientists called for an end to the practice, and prominent statisticians, including the head of the American Statistical Association, put it bluntly: "Don't say 'statistically significant.' " Too often, they said, this binary labeling of results as worthy or unworthy has become "the antithesis of thoughtfulness," a shortcut around what should be the hard work of any statistical inquiry.
What we need for the under-5 vaccine trial evaluation, instead of judgments of absolute safety or efficacy, is probable improvement over the next best alternative, taking into consideration all the available information. Even the concept of an emergency use authorization challenges the ordinary FDA binary of approval and disapproval. We should take that idea and extend it.
There is a version of statistics that would be more suitable than significance testing for evaluating this trial data: Bayesian statistics. The essential tenets of this approach are that investigators should constantly update our understanding of any scientific claim based on the latest data and that we never need to label such a claim as definitively proved or disproved.
This methodology has had successes in many domains, from sports analytics to online commerce, and it shines the most when data is limited. Bayesian methods allowed Allied cryptanalysts in World War II to break enemy ciphers using only a few intercepted messages, and similar techniques are essential to marine search-and-rescue operations working from a vessel's last known position or fragments of debris.
A Bayesian analysis of the vaccine for children under 5 would consider both that Pfizer's mRNA vaccine has an excellent track record of safety for older children (obviously a 6-month-old is not a 5-year-old, but nor are they an entirely different species) and that we can already make reasonable estimates of how effective a two-dose regimen for little children will be, even against the omicron variant. And if the newest data shows the vaccine losing effectiveness against this variant at the currently recommended dosages and schedules, statistical techniques that can incorporate this information as quickly as possible should be used to guide any necessary changes to the protocols.
The practice of borrowing information from one experiment to help understand another is not unprecedented. The FDA has acknowledged the value of a Bayesian approach in certain circumstances, including pediatric trials. A 2020 policy document states, "Bayesian inference may be appropriate in settings where it is advantageous to systematically combine multiple sources of evidence, such as extrapolation of adult data to pediatric populations." And the agency's guidance for medical device clinical trials — where Bayesian methods have been more accepted for years — includes the endorsement that "Bayesian analysis brings to bear the extra, relevant, prior information, which can help FDA make a decision." The best way to demonstrate the advantages, when the under-5 vaccine is back up for review, would be for those evaluating the vaccine to put on their Bayesian goggles and consider the whole picture.
Referring to the vaccine trials for children under 5, Dr. Gregory Poland, the founder and director of the Mayo Vaccine Research Group in Minnesota, said recently, "I don't like that there isn't more data." Neither do I and other parents. But I also don't like that my children are unvaccinated going into year three of the pandemic. If the vaccines are safe — and we know they work well in other age groups — that's meaningful to me both as a parent and as a statistician.
A 2018 editorial in the Journal of the American Medical Association suggested that when it comes to evaluating trial results, it's time for clinicians to "embrace their inner Bayesian." The same goes for the pharmaceutical industry and the agency that regulates it.
Now is the time for a statistical overhaul. If ever there was a trial that cried out for Bayesian methods, this is it. And if ever there were institutions powerful enough to bring about a fundamental change in the ways we interpret data, it would be the FDA and the pharmaceutical companies during the pandemic. In the meantime, people across the country who fret about their unvaccinated young children will continue to do what we've become experts at: waiting.
Aubrey Clayton is a mathematical statistics researcher and a parent to three children under 4. He's the author of "Bernoulli's Fallacy: Statistical Illogic and the Crisis of Modern Science." This article originally appeared in the New York Times.
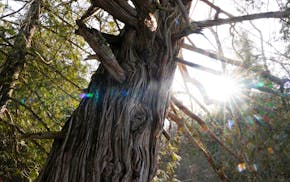
BWCA's future likely hinges on election
Saving the dinosaurs: Why there's a future for community newspapers
Readers Write: Gun storage laws, Uber and Lyft, 24/7 businesses, the pipe organ
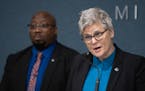